What is Retrieval Augmented Generation (RAG)? All to Know
.avif)

Do you have a project in mind?
_%20All%20to%20Know.png)
Imagine asking a question and receiving a response combining deep understanding and up-to-date information. That’s the magic of RAG AI.
But what is RAG, exactly?
Retrieval-augmented generation (RAG) is a groundbreaking approach that improves how AI models provide answers by pairing retrieval systems with generative capabilities. Instead of relying only on preexisting training data, RAG draws from diverse and updated sources to deliver accurate and contextually rich responses every time.
This blog explores the details of RAG AI, explaining how it works and why it is transforming industries such as customer support, healthcare, and legal services.
Read on!
What is RAG?
Retrieval Augmented Generation (RAG) is a method that pairs retrieval-based systems with generative AI models to improve how machines find and deliver information.
Instead of relying solely on a single database or its training data, RAG pulls in external knowledge from vast, diverse sources like vector databases, knowledge graphs, or even web pages. It then incorporates this data into responses, making them more accurate and relevant to user input.
Here’s how it works in simpler terms.
A retrieval model first searches for the most relevant documents or data points from structured and unstructured data. It uses techniques like semantic search to ensure the results align closely with a user’s query.
Once the needed information is retrieved, the generative AI model uses this input to form complete and coherent answers, combining what it retrieved with its language understanding.
This hybrid approach excels in tasks requiring much knowledge, such as answering questions, summarizing research reports, or offering solutions in fields like customer service and technical support.
Unlike traditional systems, which may produce outdated or less precise replies, RAG keeps responses current by utilizing fresh external data and relevant documents.
Organizations like Meta AI have championed this technology, exploring its use in natural language processing and enterprise-level workflows.
Some real-world applications include:
- Assisting with customer service guides, offering detailed answers and the right references.
- Improving knowledge-intensive tasks like answering complex technical or legal questions.
- Simplifying access to enterprise data while safeguarding sensitive information.
%20include_.png)
How RAG Works in Generative AI Models
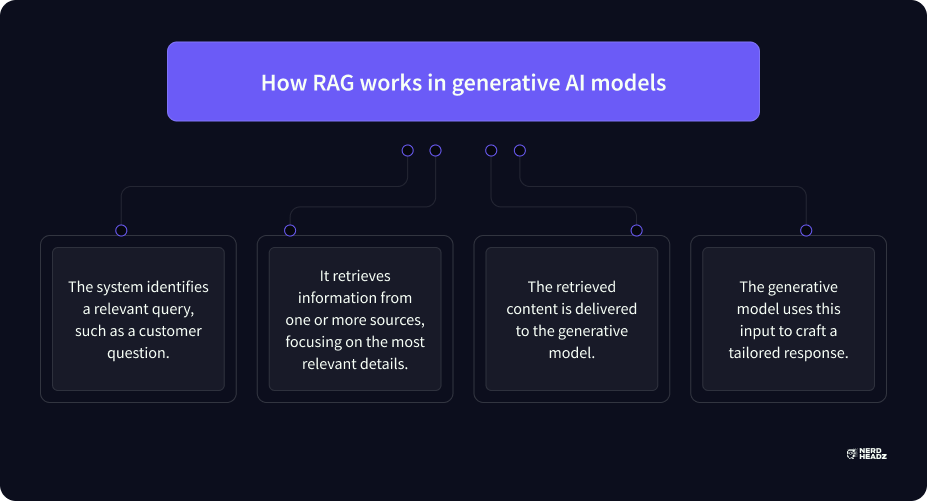
Retrieval Augmented Generation (RAG) operates on a model that blends two distinct AI capabilities into a unified process. It capitalizes on retrieval systems and generative models to respond to complex user queries with precise, comprehensive answers.
Here’s a closer look at how it works behind the scenes.
RAG integrates two components. First, the retrieval system is designed to find relevant information in structured or unstructured data. It can search across enterprise data, knowledge bases, or external repositories.
The retrieval model uses advanced techniques, such as semantic search, to ensure that the information matches the user’s input as closely as possible.
The generative model then steps in to create responses.
Based on the retrieved data, it uses advanced language capabilities to compose detailed, coherent outputs. Large language models, often trained on diverse datasets, enable this phase, allowing answers to feel natural while incorporating all the key supporting details.
A process called an “augmented prompt” facilitates this interaction.
The augmented prompt helps the generative AI contextualize retrieved details, ensuring the results address specific questions or tasks. RAG can perform knowledge-intensive work by combining retrieved and generated data, such as customer service guides or document summarization.
Here’s how the workflow unfolds in simple steps:
- The system identifies a relevant query, such as a customer question.
- It retrieves information from one or more sources, focusing on the most relevant details.
- The retrieved content is delivered to the generative model.
- The generative model uses this input to craft a tailored response.
This hybrid method reduces the chances of inaccuracies and minimizes computational and financial costs. It prioritizes external and internal resources effectively to avoid waste, making it practical for businesses handling sensitive or massive amounts of data.
RAG systems have gained attention for their ability to produce up-to-date information even when the original training data is no longer relevant. They remain reliable by connecting with external sources such as ongoing research, customer queries, and new data streams.
Applications for RAG are plentiful.
From improving enterprise workflows to improving natural language processing tasks, these systems can significantly bolster efficiency without compromising detail or accuracy.
For businesses, this might look like handling customer queries, analyzing internal knowledge, or maintaining a consistent knowledge base across various teams. With this blend of retrieval and generative capabilities, RAG offers a practical solution for today’s demand for intelligent systems.
Key Benefits of Using Retrieval Augmented Generation
Improved Accuracy of AI Outputs
Retrieval Augmented Generation (RAG) enhances the reliability of AI-generated responses by combining retrieval models with generative AI.
Instead of relying solely on the data used during training, RAG pulls relevant information from external data sources such as knowledge bases or enterprise data systems. This approach ensures that the provided information is accurate and contextually appropriate for each user query.
For instance, when responding to a technical question, RAG can include semantically relevant passages from up-to-date research or internal documents, improving the precision of the output.
Reduction in Misinformation and “Hallucination” Issues
One common challenge with some generative AI models, like large language models, is their occasional tendency to generate incorrect or misleading content, often called “hallucination.” RAG mitigates this issue by grounding the generated content in retrieved data.
By incorporating external references during the response generation phase, the system minimizes the chances of creating unsupported or fabricated information. This makes RAG particularly valuable for applications such as question-answering systems and customer service, where accuracy is critical.
Access to Real-Time and Domain-Specific Data
Traditional AI models are typically limited to the data they were trained on, which can lead to outdated results over time.
RAG solves this problem by integrating external and domain-specific sources during retrieval. It ensures that responses incorporate the latest available information for tasks requiring real-time insights, such as financial data analysis or responding to highly specialized queries.
Businesses use this sensitive data securely or use external repositories to meet the unique demands of their industries.
Cost-Efficiency Compared to Model Retraining
Large language models must be regularly retrained to stay up-to-date, which is computationally demanding and financially prohibitive. Organizations can avoid frequent retraining cycles by adopting RAG workflows.
Instead, integrating retrieval systems allows AI to access fresh, external data sources as needed.
This balance between retrieval and generative processes reduces operational costs without sacrificing the relevance of the generated responses. This cost-efficiency makes RAG an appealing solution for businesses managing vast enterprise data.
With its ability to combine accurate information retrieval and generative capabilities, RAG offers practical advantages for businesses and technical applications.
Components of RAG AI

Combining Retrieval & Generation Processes
Retrieval Augmented Generation (RAG) operates by merging two powerful AI techniques into one cohesive system.
The retrieval component captures the most relevant information from large internal or external datasets. This information is the foundation for the generative model, which uses it to produce detailed and accurate responses tailored to specific user input.
These processes complement one another rather than working in isolation.
The retrieval phase ensures the most pertinent data points are available, while the generation phase transforms that data into natural, understandable text. This cooperation reduces errors and increases the practicality of the generated results, making the technology highly effective for enterprise applications and problem-solving tasks.
The Role of Knowledge Bases & External Databases
The success of RAG AI often depends on the quality and diversity of the data sources it can access.
Knowledge bases, for example, act as repositories of structured data, such as customer profiles or product specifications. External databases, which may include web pages or research papers, add another layer of depth by providing up-to-date and domain-specific information.
When user queries demand precise answers, RAG pulls from these sources to ensure relevance and accuracy. Integrating sensitive internal data with external repositories allows the system to address various topics without compromising security or reliability.
Using Vector Databases & Semantic Search
RAG’s key strength is retrieving contextually relevant information from vast datasets.
Vector databases play a crucial role, enabling the system to store and retrieve data based on numeric representations of meanings instead of exact keyword matches.
Paired with semantic search techniques, this approach helps identify passages that align closely with a user’s query, improving the precision of retrieved results. For instance, someone asking for technical specifications might receive detailed sections from a knowledge base, even if the query’s wording doesn’t explicitly match stored data.
This capability ensures the retrieval process delivers content that aligns with user intent, allowing the generative AI model to produce responses that truly address user needs.
Applications of RAG in Various Industries
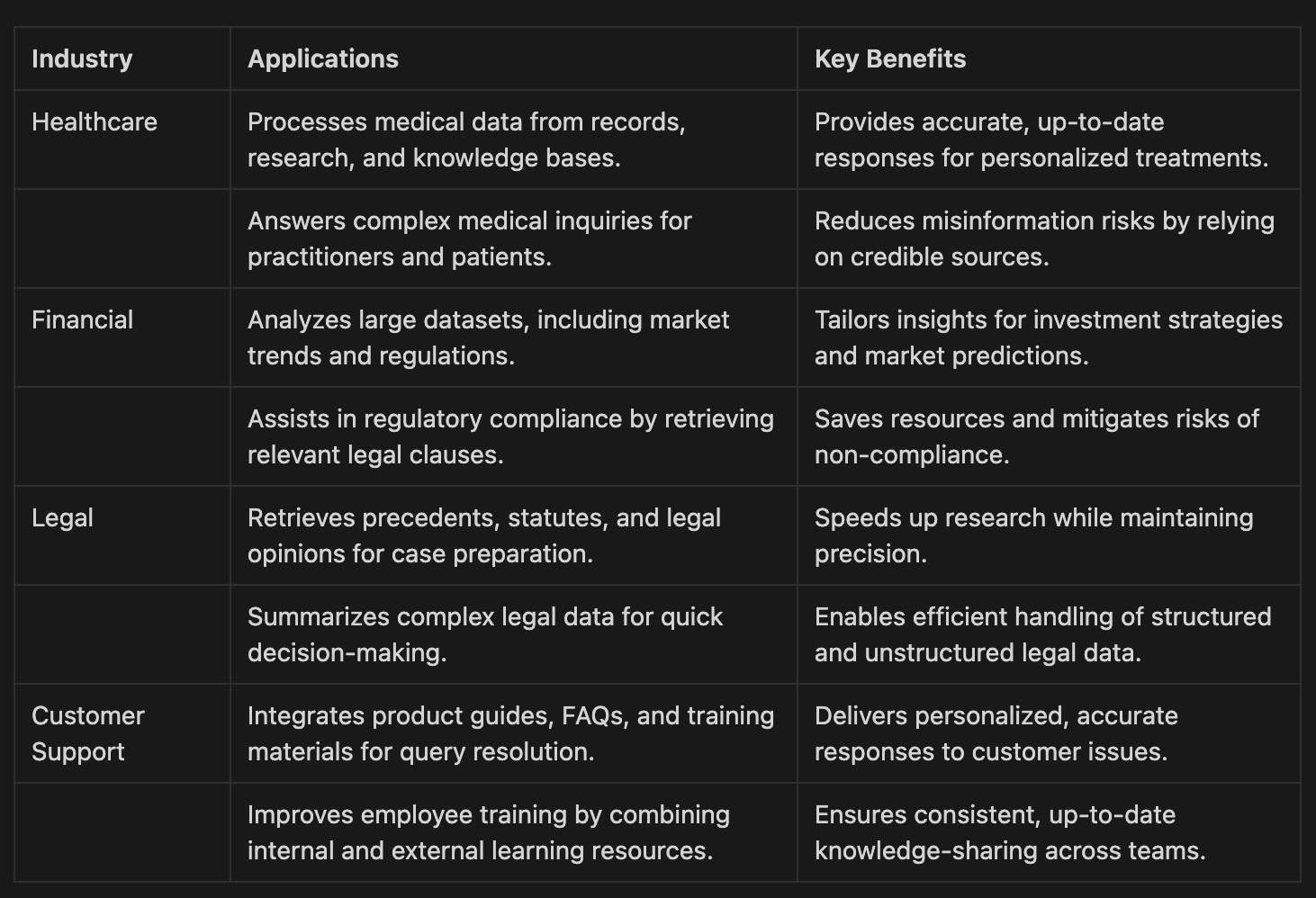
Healthcare Applications
Retrieval-augmented generation (RAG) is making significant strides in healthcare by improving the precision and accessibility of medical data handling.
With the ability to draw from multiple sources, such as electronic health records, research studies, and specialized knowledge bases, RAG provides accurate responses to complex medical inquiries. This is particularly useful for personalized treatment plans, allowing practitioners to manually consider all the data available without sifting through extensive documentation.
For example, a physician could input symptoms into a system powered by RAG AI, receiving results improved by up-to-date research or patient-specific details.
Additionally, RAG reduces the risk of misinformation when answering patients’ medical queries. Its reliance on credible, curated data sources ensures that responses meet the high reliability required in such a critical industry.
Financial Use Cases
The financial sector benefits from RAG’s ability to process and analyze enormous datasets while ensuring efficiency and relevance.
By integrating data sources like internal reports, market trends, and government regulations, RAG tailors its outputs to the needs of analysts, managers, or even individual consumers. For instance, it can synthesize detailed, real-time updates to assist in predicting market scenarios or generating insights for investment strategies.
Another key use is in regulatory compliance.
Financial institutions must keep up with constantly evolving laws and guidelines. RAG allows organizations to retrieve and apply specific clauses relevant to their activities without delay. This can save firms resources and avoid non-compliance risks.
Legal Industry
RAG AI improves efficiency within the legal field by simplifying access to precedents, statutes, and legal opinions.
It processes diverse data points using a combination of retrieval and generative techniques and presents accurate summaries or analyses tailored to specific legal inquiries. Lawyers, paralegals, or firms that leverage such tools can make decisions more quickly without compromising precision.
A strong feature of RAG in this sector is its ability to handle complex and structured data effectively.
For instance, it can retrieve the most relevant case studies using a query, enabling practitioners to focus on strategizing rather than manually performing research. This capability is invaluable for tight deadlines or high-stakes litigation.
Customer Support and Training
Using RAG for customer support adds a layer of personalization and accuracy that many traditional systems struggle to achieve.
Customer service centers can integrate RAG workflows to answer queries by pulling from product guides, FAQs, or training materials. The generative aspect then refines this retrieved information into responses specific to each customer’s issue, ensuring relevance and empathy.
Beyond solving direct customer queries, organizations can leverage RAG for employee training. Businesses enable comprehensive, up-to-date knowledge-sharing by integrating structured learning materials with external resources.
RAG’s adaptability and efficiency in this area simplify education while offering consistent and reliable knowledge-sharing outputs.
Challenges in Building and Using RAG
Managing Data Sources and Ensuring Data Quality
One of the biggest challenges in implementing Retrieval Augmented Generation (RAG) lies in managing diverse data sources while maintaining high-quality standards.
RAG systems rely on internal data and external repositories to produce relevant results. The output becomes unreliable if the input data lacks structure or contains inaccuracies. Issues like mismatched formats or missing fields can lead to gaps in the retrieval and generative processes.
To address this, organizations need robust pre-processing strategies.
Businesses can build a more reliable foundation by ensuring a consistent format and verifying the accuracy of their source data. Regular updates to databases, particularly external sources, also help keep information current.
A well-curated knowledge base ensures that the content retrieved is accurate and applicable to user queries, increasing the quality of the response.
Avoiding Over-Reliance on Retrieved Data
Relying too heavily on the retrieval phase may result in incomplete or misleading outputs, especially if the data sources aren’t comprehensive.
For example, limited or outdated content may cause the generative AI to produce results that fail to address complex or niche user needs. This challenge is amplified when dealing with sensitive data or highly specific domains, such as healthcare or legal industries.
Designing checks and balances within the RAG architecture to mitigate such risks is crucial.
Incorporating multiple retrieval methods, such as keyword and semantic search, boosts the chances of retrieving semantically relevant passages.
Beyond that, combining retrieved insights with contextual understanding drawn from the language model can lead to more effective responses that meet user expectations. Regularly reviewing the system’s workflows ensures proper internal and external data integration without losing reliability.
Balancing Performance with Scalability
Scaling RAG systems to handle large volumes of queries and data presents another challenge.
High response rates are often prioritized in enterprise applications, but without efficient scalability strategies, performance can suffer. Large language models used in RAG may consume significant computational resources, particularly when processing vast quantities of structured and unstructured data.
Finding the right balance requires thoughtful hardware and software optimization.
Vector databases play a significant role in improving the efficiency of retrieval processes. These databases store data as numerical representations rather than text, enabling faster and more accurate search results even at scale.
Similarly, fine-tuning the retrieval and generative models helps the system adapt while maintaining high performance. Adopting a modular approach to RAG implementation can also allow for focused improvements on specific system components without disrupting the workflow.
What Does The Future Hold of Retrieval Augmented Generation
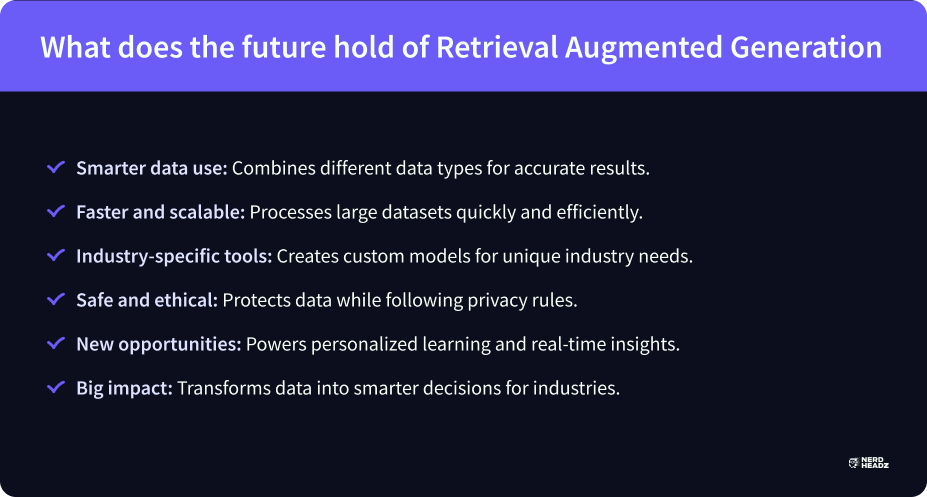
Retrieval Augmented Generation (RAG) is quickly establishing itself as a game-changer in artificial intelligence applications, paving the way for exciting advancements across various fields.
RAG’s future seems promising, driven by continuous improvements in its architecture and the increasing demand for intelligent systems.
One of the most anticipated progressions in RAG AI is refining how systems interact with diverse data sources. Future implementations will likely integrate more robust mechanisms for managing structured and unstructured data, resulting in higher precision within generated responses.
RAG systems will better address complex user requirements across expanding industries by incorporating up-to-date information from multiple repositories.
Experts continue to focus on designing systems capable of managing larger datasets while maintaining speed and relevance.
Efforts to optimize RAG architecture include adopting advanced machine-learning techniques and facilitating seamless integration with vector databases. This will allow organizations to process and retrieve more meaningful data representations without sacrificing computational speed.
Improved workflows supporting real-time responses will improve user experience and productivity, especially in high-demand environments like customer support centers and global enterprises.
Another exciting possibility lies in the development of domain-focused RAG systems.
Future enhancements may include tailored models catering to industries or niche applications. For instance, healthcare-oriented RAG could prioritize secure access to clinical data, while legal applications might adopt precise contextual case law retrieval mechanisms.
Ethics and data security remain top priorities as RAG technology grows.
Future advancements will aim to protect sensitive data during processing without compromising system functionality. More transparent and accountable practices could also emerge, ensuring retrieval augmented generation work adheres to strict regulations while respecting user privacy.
RAG AI’s future promises to change how data becomes actionable intelligence.
Focusing on scalability, security, and adaptability, these systems are poised to make significant strides in meeting real-world challenges. Whether helping doctors provide personalized care or enabling business leaders to make informed decisions, the Retrieval-Augmented Generation will likely remain at the forefront of innovation for years.
NerdHeadz Can Build Your RAG Project
Retrieval-augmented generation (RAG) has emerged as a powerful approach to AI technology, and NerdHeadz, a Clutch Global Web Development Leader, is here to help you take advantage of it.
With a strong focus on understanding your needs, NerdHeadz ensures that every RAG AI project is tailored to meet unique requirements. We integrate advanced techniques like retrieval and generative models, helping businesses use real-time data for smarter, context-aware solutions.
From managing diverse data sources to delivering efficient solutions, NerdHeadz knows how to handle the complexities of a RAG model. Our team creates robust systems capable of retrieving related data quickly while maintaining accuracy.
Here’s what sets NerdHeadz apart:
- Custom solutions: We work closely with clients to design models that align with specific needs, improving outcomes for different sectors.
- Data expertise: We effectively manage and integrate new data into RAG systems without compromising response quality.
- Scalability: Their solutions are built to handle growing datasets and maintain performance under increased usage.
RAG AI can potentially turn raw information into meaningful, accessible intelligence. With the expertise of NerdHeadz, you don’t just build a project; you create a competitive edge.
Conclusion
Retrieval-augmented generation (RAG) has proven to be a powerful method for improving AI’s interaction with data. RAG ensures accurate, relevant, and context-aware responses by combining robust retrieval systems with generative AI.
Unlike traditional models, it draws from dynamic and diverse data sources, offering a reliable solution for complex tasks such as customer support, legal research, and healthcare applications.
Understanding RAG and how RAG AI works opens up opportunities to optimize decision-making, reduce misinformation, and improve operational efficiency. Whether you’re managing large datasets or tackling knowledge-intensive tasks, RAG provides a scalable and effective solution tailored to modern needs.
If you’re ready to see how RAG can transform your processes, NerdHeadz is here to help.
Reach out to explore tailored RAG AI solutions that simplify workflows and unlock new productivity levels.
Frequently asked questions
What is a RAG used for?
Retrieval-augmented generation (RAG) creates AI models that retrieve real-time data and generate accurate, context-aware responses. It’s especially useful for industries where up-to-date information is critical, such as customer support or research.
What is RAG with example?
RAG combines AI retrieval systems and generative models to provide relevant information. For example, a RAG-powered chatbot could pull real-time policy updates from a database and generate responses tailored to customer queries.
What does RAG stand for?
RAG stands for Retrieval Augmented Generation, an AI method that improves outputs by combining data retrieval and text generation.
What is the difference between semantic search and RAG?
Semantic search retrieves results based on meaning, while RAG uses those results to generate contextually rich, customized responses through AI.