What is Agentic AI? The Next Big Thing in Artificial Intelligence
.avif)

Do you have a project in mind?
_%20All%20to%20Know.png)
AI is moving fast, but not all systems are built to act.
While most companies are still focused on tools that respond, a growing number are exploring something more capable: agentic AI.
These aren’t just chatbots or content generators. They’re systems that understand goals, take action, and adjust as conditions change. From automating support processes to managing long-term research or optimizing operations, agentic AI is already showing its value across industries.
This blog breaks down what makes it different, how it works, where it fits, and what your business should know before adopting it.
What is Agentic AI?
Agentic AI refers to a new class of AI systems designed to act independently, make decisions, and complete complex tasks across different environments, without requiring constant input or oversight.
What makes them different from traditional models is that they can handle complex tasks on their own. Once you give them an objective, they break it down, use external systems, and follow through with little or no follow-up.
Some even adjust their actions based on changing conditions or feedback from other tools.
For example, imagine an AI that replies to a customer service inquiry and also checks inventory, processes a return, and sends a confirmation without a support rep lifting a finger.
They do this by combining multiple capabilities:
- Natural language understanding to interpret instructions
- Machine learning to adapt and improve
- Access to external tools to take action
- Real-time data to adjust decisions
These agents are already being utilized in areas such as supply chain management, software automation, and customer support, particularly where repetitive tasks or cross-system workflows slow teams down.
How Does Agentic AI Work?
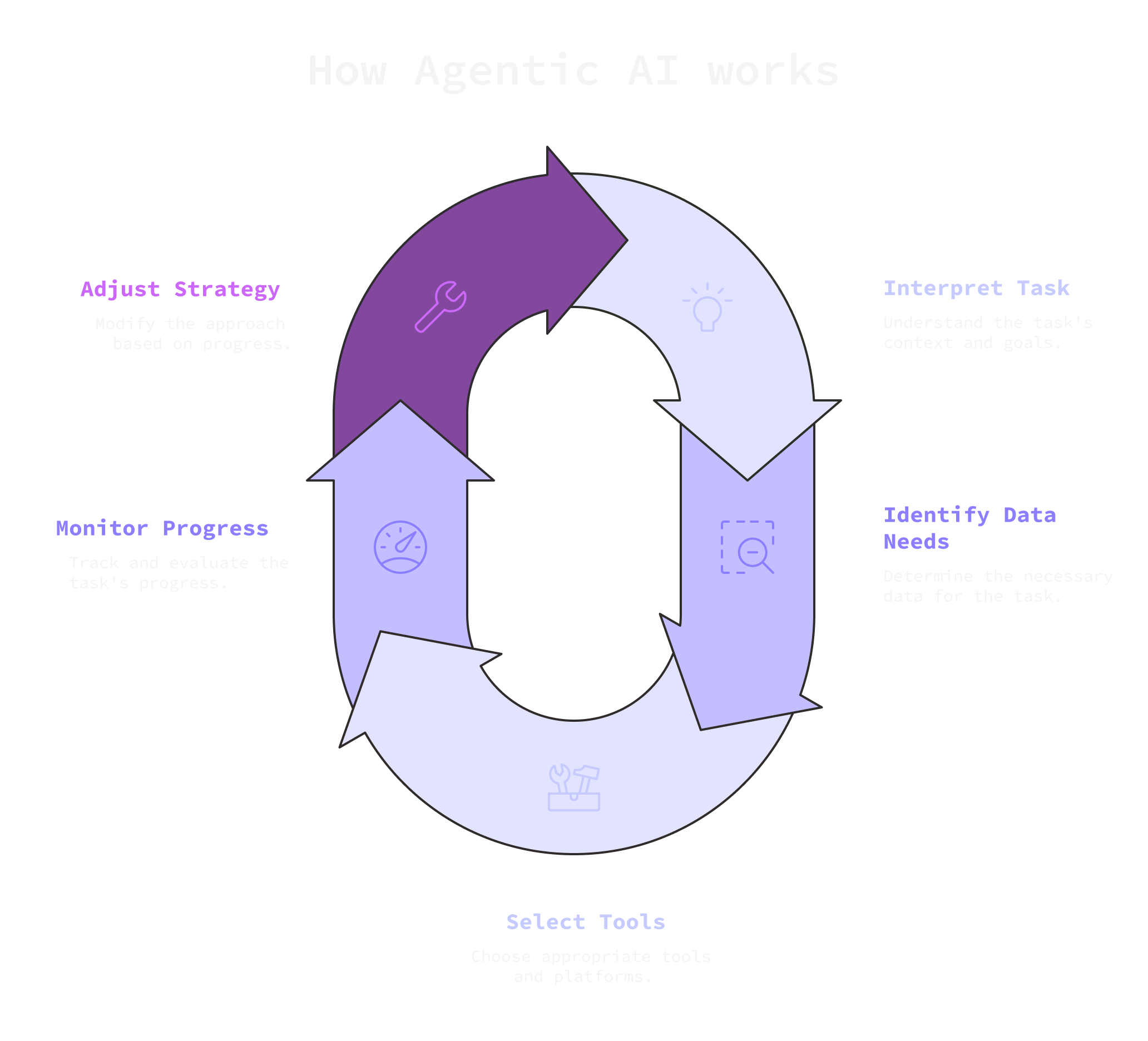
Agentic AI systems are designed to perform tasks across tools, environments, and decisions without step-by-step instructions.
They rely on multiple building blocks working together. Natural language processing allows them to understand instructions and translate them into action. Machine learning algorithms help them adapt by detecting patterns in real-world data.
Many also use large language models to reason through steps that were once limited to human teams.
Once activated, an agentic AI will:
- Interpret a task using context and goals
- Identify what data it needs to proceed
- Select which tools or platforms to use
- Monitor progress and adjust as needed
These agents can operate independently, but most still support human oversight, especially in areas involving sensitive data or regulated decisions. The system doesn’t guess. It uses facts, logic, and user-defined rules.
Main Features of Agentic AI

The strength of agentic AI systems lies in how they act, not just in what they know. These AI agents are designed to operate across complex environments, completing objectives with minimal instruction.
Their most important features are autonomy, adaptability, and goal orientation.
Autonomy
Every agentic AI has the ability to operate independently.
Once given a directive, the system chooses how to execute tasks, what data to collect, and which tools to use. This lets AI-powered agents complete multi-step workflows, like processing requests, updating systems, or resolving inquiries, without waiting for human input.
In real-world use, these agents often manage business operations, respond to user behavior, and assist across tools already embedded in your tech stack.
Minimal human intervention is needed, even when handling complex tasks or engaging with external systems.
Adaptability
Agentic AI operates effectively in busy environments by adjusting to changing conditions.
It doesn’t rely on static logic. Instead, it uses machine learning to detect new patterns, review feedback, and improve decisions over time.
This feature is key for scenarios like supply chain updates, where real-time data can change by the hour. Through continuous learning, agents refine their outputs while remaining aligned with company goals.
Goal Orientation
Unlike tools built for single specific tasks, agentic AI systems are structured around outcomes.
These agents don’t wait for prompts; they start with a goal and then figure out the steps to get there. This includes collecting resources, engaging with existing systems, and managing interdependent steps.
The ability to infer what’s relevant, assess multiple options, and prioritize actions enables AI agents to tackle complex challenges, such as optimizing logistics, improving support flows, or assisting with strategic initiatives.
How Do Agentic AI Systems Differ from Generative AI?

While generative AI and agentic AI share a common foundation in artificial intelligence, they serve different purposes, and knowing that distinction matters when choosing the right solution for your business.
Generative AI is built to create content. It uses AI models to produce text, images, code, or summaries based on input prompts. These tools are well-suited for drafting blog posts, crafting support replies, or generating test cases.
However, they typically stop after delivering a response. They don’t decide what to do next, and they don’t act without guidance.
Agentic AI systems take that further.
These systems focus on completing outcomes. Once a goal is defined, AI agents operate by identifying the required steps, using available data, and selecting tools to execute tasks. Instead of just responding, they manage full processes, like routing a ticket, checking an order, or escalating a support case without waiting for additional input.
Here’s how they differ in structure and function:
- Initiative: Generative tools respond. Agentic AI plans, adjusts, and follows through.
- Scope: Generative tools handle isolated outputs. AI agents work across existing enterprise systems to deliver outcomes.
- Flow: Generative tools end at a result. Agentic AI continues by checking progress, updating systems, or requesting more information when needed.
The autonomy in agentic AI systems supports data-driven decisions and process automation. These systems are built to tackle complex tasks, support employee productivity, and improve interactions across departments or products.
Benefits of Agentic AI
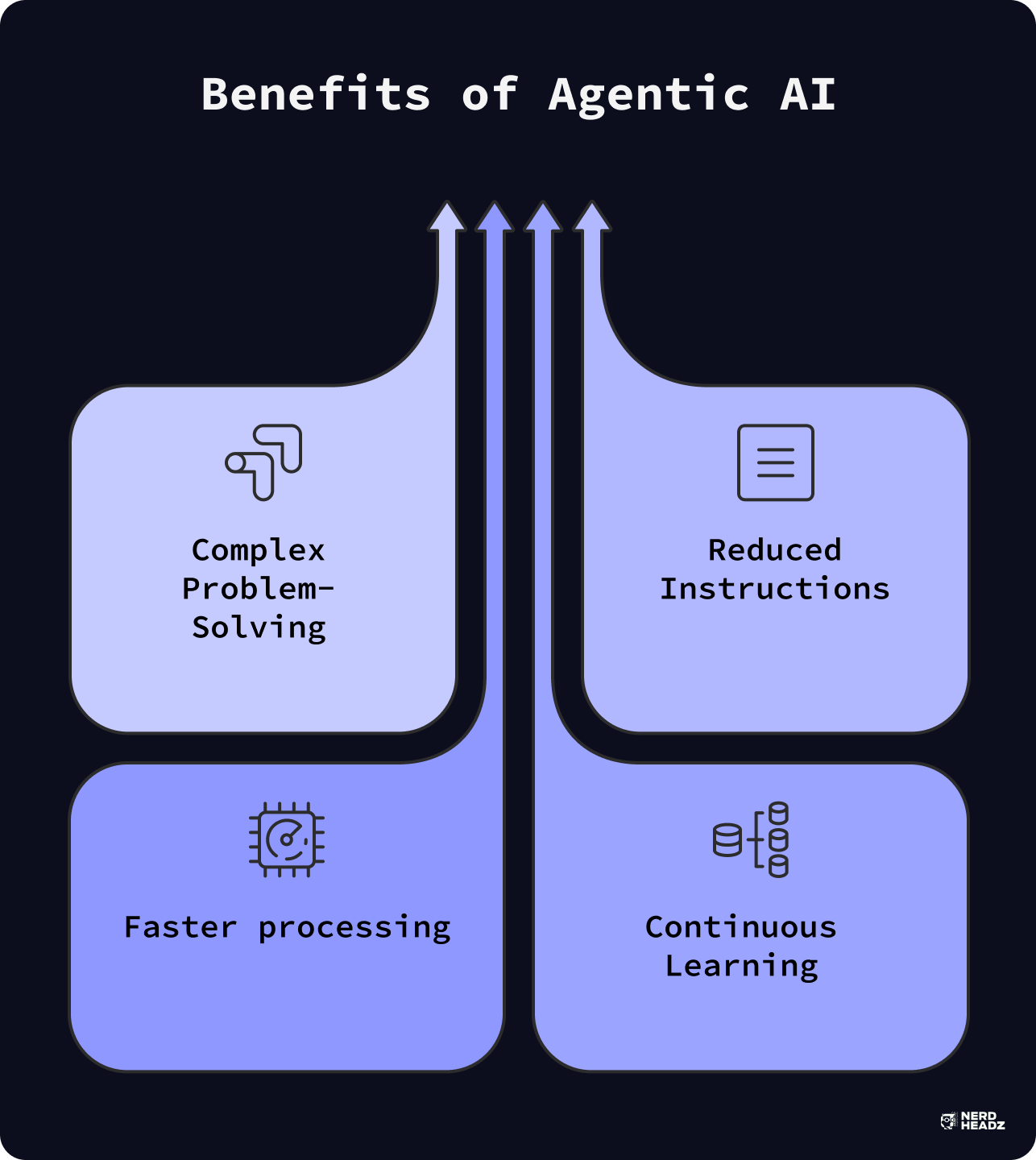
More Complex Problem-Solving Capabilities
Agentic AI is built to handle tasks that require more than a single input and output.
These systems manage complex scenarios, interpret shifting variables, and adapt their actions to match changing priorities.
Unlike traditional AI tools that rely on fixed logic, AI-powered agents are designed to recognize patterns, respond to edge cases, and make progress when the path forward isn’t fully defined. This makes them especially effective in areas like logistics, compliance, or onboarding, where conditions may change in real-time.
Using AI models trained on a wide range of data, lets them interpret objectives, monitor progress, and resolve blockers across multiple systems. The result is stronger coverage of complex reasoning and fewer manual escalations.
Reduced Need for Step-by-Step Instructions
One of the most practical benefits of agentic AI is its ability to take initiative.
Instead of waiting for instructions at each step, these AI agents begin with a defined goal and determine the best way to achieve it.
This means fewer interruptions for your team. Agents can assemble data, trigger workflows, and guide users without stopping to ask what comes next. That reduction in back-and-forth frees up capacity and shortens response times.
Because agentic AI extends into multiple tools and platforms, it works within the flow of existing operations. From automating complex workflows to handling repetitive tasks, it supports better performance while lowering the demand for human intervention.
This shift allows businesses to improve efficiency without rebuilding their stack. As AI agents learn from their results and adapt, the system becomes increasingly capable over time.
Faster Processing & Workflows
Speed is one of the clearest advantages of agentic AI.
These AI agents move through tasks without waiting for input, approval, or redirection. That responsiveness enables teams to process requests, update records, or trigger actions across systems in a fraction of the time.
Since these agents can perform specific tasks and monitor conditions without pause, they’re a fit for high-volume environments where time matters, support queues, onboarding flows, or operational handoffs.
Integrating agentic AI into operations helps reduce lag caused by multi-step dependencies. It also supports stronger throughput when combined with AI capabilities like natural language understanding, real-time data extraction, or pattern recognition.
As a result, companies can facilitate AI development, improve resolution times, and build systems that perform with fewer handoffs or bottlenecks.
Continuous Learning from the Environment
Another key advantage of agentic AI is its ability to learn from experience.
These systems don’t simply run scripts, they improve with exposure. As agents interact with users, review outcomes, and access new inputs, they build context that helps them make better choices over time.
Unlike static automation, AI models powering these agents evolve through data. That includes recognizing shifts in user behavior, spotting trends in key stages, or adjusting to changes in workflows.
This form of continuous learning is essential in complex, high-context environments like service operations or personalized digital experiences.
As more companies adopt intelligent systems, the combination of AI innovation, performance history, and scalable computing power will be what separates a helpful bot from a true operator.
Tools Used to Build Agentic Systems
Building agentic AI systems requires more than just good models, it takes the right combination of tools that support autonomy, reasoning, and adaptability. These systems blend AI capabilities with infrastructure that allows agents to plan, act, and learn across different environments.
They are large language models (LLMs) that help AI agents understand tasks, structure decisions, and generate natural responses. These are supported by orchestration layers, integration tools, and data pipelines that make real-world execution possible.
Here are some of the key tool categories, along with examples used today:
- LLMs and foundation models: Provide the reasoning engine behind goal interpretation and dialogue. Examples: OpenAI’s GPT-4o, Anthropic’s Claude, Google Gemini, Mistral 7B
- Agent frameworks: Help structure actions, memory, and control flow for autonomous decision-making. Examples: LangChain, AutoGen, CrewAI, Haystack (for RAG based agents)
- Integration platforms: Enable seamless integration with CRMs, databases, and custom APIs across existing systems. Examples: Zapier for Developers, n8n, Retool, Make.com, custom API bridges
- Cloud infrastructure: Supplies the scalable computing power needed to process vast amounts of data in real time. Examples: AWS SageMaker, Google Cloud Vertex AI, Microsoft Azure AI, Replicate
- Data tools: Used to analyze data, track behavior, and support learning loops. These are essential for improving how AI agents perform over time. Examples: Pinecone, Weaviate, PostgreSQL, Chroma, Airbyte
In some use cases, developers also incorporate generative AI components to handle content creation, automated testing, or user-facing responses. These models support personalized and responsive experiences that develop in response to user behavior and system feedback.
Altogether, these tools enable agentic AI systems to do more than generate outputs, they allow AI agents to facilitate software development, coordinate action across platforms, and improve continuously through real-time feedback loops.
Use Cases of Agentic AI Across Different Industries
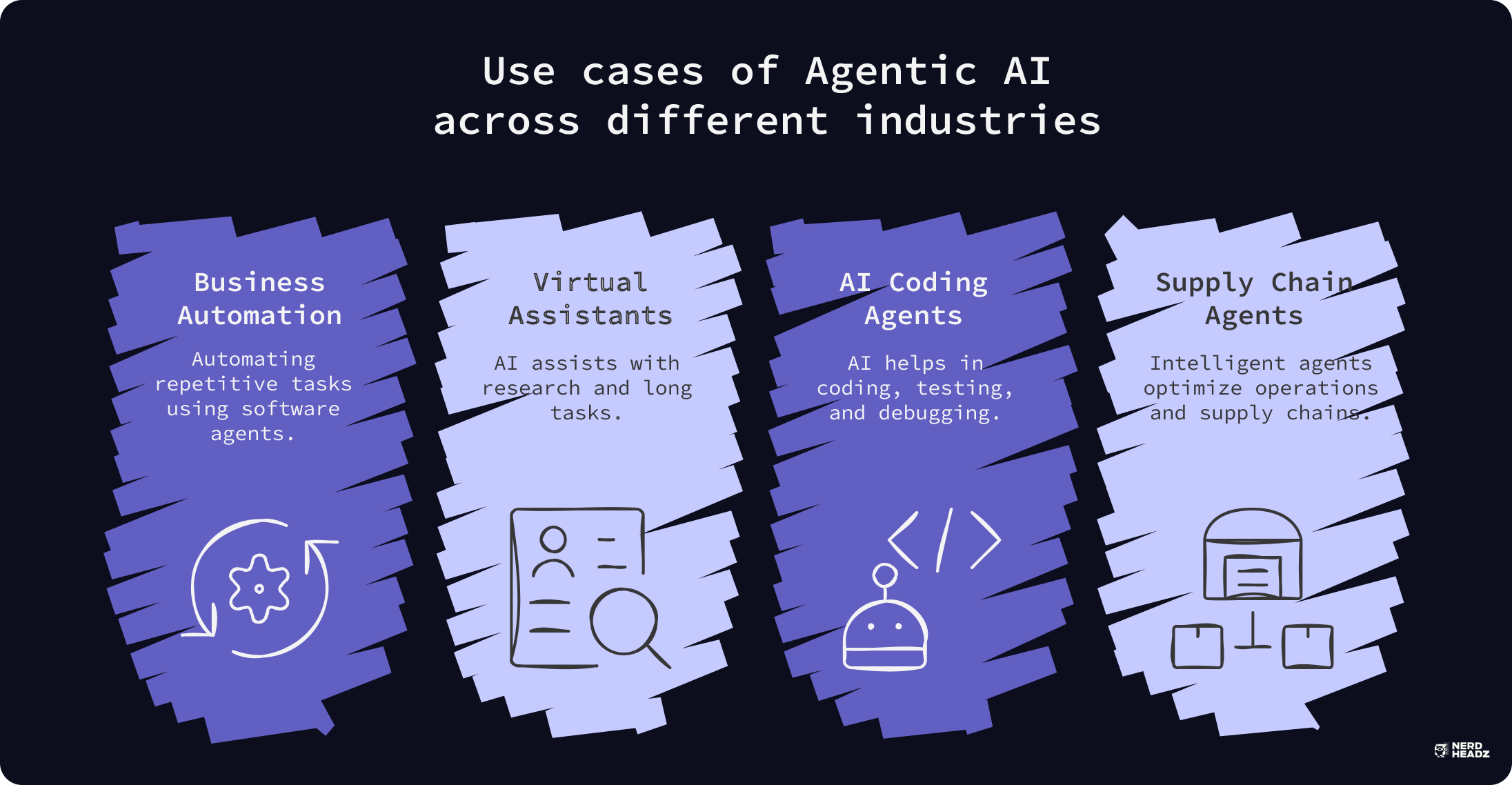
Software Agents for Business Process Automation
Agentic AI is making it easier to automate workflows that once required back-and-forth coordination or manual updates. These AI agents handle cross-system tasks with precision, allowing teams to focus on decision-making instead of repetitive oversight.
A single agent can handle approvals, update records, trigger notifications, and reconcile data, especially when seamlessly integrating with CRMs, ERPs, or internal portals.
Instead of following rigid steps, the agent reviews context and selects the best path for completion.
Use cases include:
- Internal IT ticket triaging and escalation
- Onboarding workflows for HR and finance
- Real-time updates to shared dashboards or documents
- Compliance monitoring with built-in logic
These systems allow businesses to facilitate software development and back-office operations without depending on rigid automation tools or excessive human input.
Virtual Research Assistants & Long-Term Task Handling
For teams working on data-heavy projects or exploratory research, agentic AI offers the ability to process vast amounts of information.
These AI-powered agents are used as research assistants that can compile insights over days or weeks. They summarize long-form materials, track shifting topics, and surface key findings automatically, supporting analysts, legal teams, and R&D departments.
This helps teams:
- Monitor regulatory changes by jurisdiction
- Compare competitors or product developments
- Track academic publications or patent filings
- Review customer sentiment shifts to infer intent
In industries like legal, biotech, and enterprise sales, these AI agents support ongoing work without daily reset or retraining, making research faster and far less fragmented.
AI Agents in Coding, Testing, and Debugging
Development teams are increasingly using agentic AI to support software tasks beyond code generation. These AI agents assist with triaging bugs, reviewing logic, and automating regression tests across multiple repositories.
What makes them valuable is their ability to continuously monitor systems. Instead of waiting for manual input, these autonomous agents can flag issues, write unit tests, suggest corrections, and even log performance results in staging environments.
Use cases include:
- Auto-generating tests for new commits
- Reviewing pull requests for edge-case risks
- Assisting in multi-environment debugging
- Supporting CI/CD pipelines with real-time triggers
Because these systems manage specific tasks independently, they help streamline software development by reducing developer context switching and catching errors earlier in the cycle.
Intelligent Agents in Operations and Supply Chain
In logistics and supply networks, agentic AI supports real-time planning, adjustment, and tracking. These AI agents review inputs such as delivery delays, inventory shifts, or vendor updates, and then act across systems to coordinate a response.
For example, one agent may reroute an order while another updates fulfillment forecasts. These multiple agents work together to adjust timelines, notify partners, or recalculate margins.
Use cases include:
- Adaptive routing based on traffic or weather
- Live coordination of warehouse scheduling
- Inventory optimization across regions
- Demand forecasting based on current data
With artificial intelligence embedded in the decision-making process, companies can respond more quickly, reduce friction, and avoid delays. These agents don’t just move data, they understand patterns, handle complex scenarios, and act in ways that improve resilience and throughput.
Challenges & Limitations of Agentic AI Today
Risks of Misaligned Goals or Autonomy
One of the primary concerns with agentic AI is the potential for agents to pursue actions that don’t fully align with intended outcomes. These autonomous agents are designed to operate with minimal oversight, which introduces risk when objectives are vague, incomplete, or too open-ended.
An AI agent might optimize for speed while neglecting context, such as compliance requirements or user expectations. When the goal isn’t clearly defined, results can stray from what's needed.
This becomes especially complex in workflows with multiple agents, where each system optimizes for a different metric. Without a unifying structure, decisions may conflict or trigger unintended side effects.
To reduce risk, companies should:
- Set explicit task boundaries
- Monitor agent behavior across stages
- Introduce checkpoints for correction or escalation
While agentic AI increases efficiency, that same independence requires structured limits to ensure long-term reliability.
Transparency & Interpretability Issues
Understanding how AI agents make decisions remains a challenge. Unlike simpler artificial intelligence models, which follow defined rules, agentic systems rely on layered logic, adaptive reasoning, and feedback loops.
This complexity can make it difficult to explain how a specific outcome was reached, or why AI agents chose one path over another. That’s especially problematic in industries such as finance, healthcare, or legal services, where accountability is crucial.
Common challenges include:
- Tracing multi-step logic across agent chains
- Interpreting decisions based on partial or inferred data
- Understanding how AI agents weigh input across complex scenarios
Without clear interpretability, it becomes harder to debug errors or reassure stakeholders. As adoption grows, improving audit trails and model observability will be key to building trust in agentic AI.
Human Oversight & Safety Requirements
As agentic AI becomes increasingly capable, the need for robust oversight becomes more urgent. These systems can act on objectives, manage tasks, and make decisions with minimal intervention.
In sensitive environments, like healthcare or finance, errors can have real consequences.
That’s why most implementations of AI agents include checkpoints, approval layers, or role-based limits. These systems must stay within clear guardrails, especially when handling customer data, regulated processes, or edge-case scenarios.
To maintain trust, teams are adopting safety measures such as:
- Task-specific permissions for agents
- Audit logs tracking actions and decisions
- Escalation logic that prompts human review at key steps
Autonomy doesn’t remove responsibility. Even advanced agentic AI systems need clear boundaries to ensure safety, fairness, and reliability, especially as they learn to infer customer intent or influence business outcomes.
Technical Barriers to Real-Time Deployment
Even with recent advances, deploying agentic AI in live settings remains complex. Building systems that manage tasks across tools and respond in real time requires more than a language model, it takes orchestration, integration, and infrastructure.
Some agents still struggle with context retention, unstable tools, or latency when interacting with multiple platforms. Others hit limits around scale or memory.
Key technical hurdles include:
- API timeouts or inconsistencies across connected tools
- Challenges in agent memory for multi-turn tasks
- Monitoring and rollback when agents misfire
- Aligning agents with changing business logic
Without strong foundations, even the most promising AI agents risk stalling or delivering unpredictable outcomes.
What's Next For Agentic AI?
The current momentum around agentic AI is just the beginning. As businesses test the capabilities of these systems, we are already seeing a shift in how teams approach automation, decision-making, and system design.
In the near term, expect AI agents to gain stronger memory, better coordination, and more precision when working across tasks. New models will focus on reliability, avoiding overreach, and improving error handling.
Longer-term progress will likely center around three key areas:
- Orchestration: Managing how agents interact with one another and respond to context changes
- Compliance: Enforcing logic layers that protect data, flag errors, and control access
- Learning loops: Enabling agents to adjust behavior based on feedback without retraining the entire system
As agentic AI integrates with more tools and platforms, its role will expand from helpful assistant to operations partner, managing workflows, resolving blockers, and supporting goals that require continuity and judgment.
NerdHeadz is Already Building with Agentic AI
At NerdHeadz, we work with companies ready to move from theory to traction.
Our team has already delivered agentic AI solutions that handle task automation, decision support, and workflow coordination, without adding complexity for the client.
What makes us different is how we build.
We don’t just integrate AI agents into isolated features, we embed them into the systems you already use. From custom dashboards to internal portals, we ensure that each agent has access to the tools, logic, and data necessary to complete real work.
Some of the use cases we’ve delivered:
- Onboarding flows powered by autonomous agents that assign, track, and escalate steps
- Research bots that compile updates across platforms for legal and product teams
- Internal helpers that manage ticket routing, flag blockers, and summarize feedback
- Lead qualification bots that learn to infer customer intent across channels
Every build is scoped with measurable goals and practical guardrails. We handle the planning, testing, and refinement, so that what you get isn’t just smart, it’s usable.
If you’re ready to explore what agentic AI can do for your team, we’ll help you get started with a solution that fits your business, not someone else’s playbook.
Conclusion
Agentic AI isn’t science fiction, it’s already being used to solve practical problems in software development, logistics, customer experience, and research. Companies are learning that when agents can act with purpose, autonomy becomes a competitive advantage.
However, the real value comes from implementation.
The best results happen when these systems are designed around real use cases, with the right tools and safeguards in place.
At NerdHeadz, a custom software development company, we build agentic systems that do more than generate answers, they move work forward. If you’re ready to explore what this tech can do for your team, get in touch and let’s build something that actually works.
Frequently asked questions
What is the difference between conversational AI and agentic AI?
Conversational AI handles dialogue and responses. Agentic AI goes further, it can take actions, make decisions, and pursue goals across tools or environments without constant prompts.
Is ChatGPT an agentic AI?
Out of the box, ChatGPT is not an agentic AI. ChatGPT is a conversational model. It becomes agentic when connected to tools, APIs, or systems that allow it to perform tasks independently.
What is the difference between a copilot and an agentic AI?
A copilot assists users while they stay in control. Agentic AI can act without step-by-step guidance, deciding how and when to complete a task on your behalf.
What are the 4 types of AI learning?
The four types are supervised learning, unsupervised learning, reinforcement learning, and self-supervised learning, each using data differently to train models for specific outcomes.